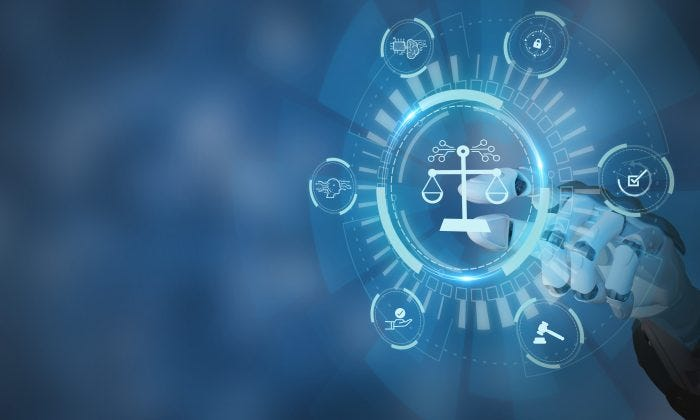
Introduction
As data science continues to evolve and permeate various aspects of daily life, the importance of ethical considerations in this field has never been more important. In 2024, data scientists are increasingly grappling with issues of bias and privacy, which can profoundly impact individuals and society. This article explores these pressing concerns and offers insights into how the learning from an inclusive data science course can help professionals address them responsibly.
Understanding Bias in Data Science
Bias in data science can manifest in several ways, affecting the accuracy and fairness of predictions, decisions, and recommendations. At its core, bias refers to systematic errors that skew data and models, often reflecting or amplifying existing inequalities. Bias can be introduced at various stages of the data science pipeline, including data collection, data processing, and model training.
A practice-oriented course in data technologies such as a data science course in Kolkata that includes several hands-on project assignments will create awareness among learners of the different types of bias that can affect data science projects:
- Sampling Bias: When the data sample does not accurately represent the population, predictions and insights may be skewed. For instance, if a healthcare model is trained predominantly on data from a specific demographic, its recommendations may not be applicable to other groups.
- Algorithmic Bias: Even if data is unbiased, the algorithms used can still introduce bias. Machine learning models often reflect the biases of their creators or training data. For example, facial recognition systems have been shown to have higher error rates for certain racial groups due to biased training data.
- Confirmation Bias: This occurs when data scientists unintentionally seek out data that supports their preconceived notions. As a result, models may reinforce existing stereotypes or patterns, rather than providing objective insights.
Addressing Bias: Steps Toward Ethical Data Science
To minimise bias, data scientists must adopt a multi-faceted approach. Here are some sure steps for ensuring ethical usage of data science as will be covered in an up-to-date data science course:
- Diverse and Representative Data: Ensuring that data sets are diverse and representative of the populations they aim to serve is crucial. Data scientists should seek to identify and fill any gaps in the data, focusing on underrepresented groups. In 2024, organisations are investing in tools and methodologies that help identify biases in data collection and preparation processes.
- Bias Audits: Regular bias audits can help organisations identify and address bias in their algorithms and data. By using tools such as fairness metrics, data scientists can quantitatively assess the fairness of their models and make adjustments as necessary. Furthermore, independent audits and third-party assessments can provide an objective perspective, helping organisations uncover biases that may not be immediately apparent.
- Interdisciplinary Teams: Bias is not solely a technical issue; it often has social and cultural dimensions. By involving experts from diverse backgrounds, including ethics, sociology, and law, data science teams can gain a broader understanding of potential biases and develop more comprehensive solutions. This interdisciplinary approach can help prevent biases that may be overlooked by a homogenous team.
- Transparent and Explainable AI: In 2024, there is an increased emphasis on making AI models transparent and explainable. By using techniques such as LIME (Local Interpretable Model-Agnostic Explanations) and SHAP (SHapley Additive exPlanations), data scientists can provide insights into how models make decisions. This transparency allows stakeholders to better understand potential biases and make more informed decisions about the use of AI.
Privacy Concerns in Data Science
In an era where data is fuelling almost all businesses, privacy has surfaced as a critical concern. Data science relies heavily on vast amounts of data, often collected from individuals who may not fully understand how their information will be used. This raises several ethical issues related to data privacy, including consent, data security, and the potential for misuse. A well-rounded data science course, irrespective of the domain it is tailored for or the level of learning it addresses, must be create awareness among students in this regard.
- Informed Consent: Obtaining informed consent is a fundamental ethical principle in data science. Individuals should be made aware of how their data will be used and given the opportunity to opt out if they wish. However, in 2024, obtaining genuine informed consent remains challenging, as individuals may not fully understand the implications of data collection and processing.
- Data Minimisation: The principle of data minimisation advocates for collecting only the data that is strictly necessary for a specific purpose. By limiting data collection, organisations can reduce the risk of privacy violations and minimise the potential impact of data breaches.
- Data Anonymisation and Pseudonymisation: To protect privacy, data scientists can use techniques such as anonymisation and pseudonymisation. Anonymisation involves removing personally identifiable information (PII) from data sets, making it difficult to trace data back to individuals. Pseudonymisation replaces PII with pseudonyms, allowing for re-identification under specific circumstances. However, as re-identification techniques become more sophisticated, ensuring true anonymity has become increasingly difficult.
Privacy in the Age of AI and IoT
The proliferation of artificial intelligence (AI) and the Internet of Things (IoT) has added new complexities to the issue of privacy. AI systems often require access to large volumes of data, including sensitive personal information. Meanwhile, IoT devices collect data from various sources, often without individuals’ knowledge or consent. In 2024, data scientists face the challenge of balancing the benefits of AI and IoT with the need to protect individual privacy. An up-to-date data science course in Kolkata and such cities where technical courses are frequently updated for addressing emerging technologies as well as for addressing any concerns reported, will orient learners to strike this balance. Here are some ways of doing this:
- Privacy by Design: Organisations are adopting a privacy-by-design approach, which integrates privacy considerations into the development process from the outset. By proactively addressing privacy concerns, data scientists can help ensure that AI and IoT systems respect individuals’ rights and comply with data protection regulations.
- Federated Learning: Federated learning is a technique that allows AI models to be trained on decentralised data, reducing the need to share raw data across networks. By keeping data on local devices and only sharing model updates, federated learning can help protect privacy while still enabling data-driven insights.
- Regulatory Compliance: In 2024, regulations such as the GDPR (General Data Protection Regulation) in the European Union and the CCPA (California Consumer Privacy Act) in the United States continue to shape data privacy practices. Data scientists must stay informed about regulatory requirements and ensure that their work complies with relevant laws.
Summary: Ethical Data Science in 2024
To address the ethical challenges of bias and privacy, a data science course must be so tuned as to imbibe a mindset of continuous improvement and accountability among data professionals. By staying informed about best practices and emerging technologies, they can develop solutions that are both innovative and ethical. Moreover, fostering a culture of ethics within organisations is essential for ensuring that data science serves the greater good.
In 2024, the stakes are higher than ever. As data science becomes more integral to decision-making across industries, addressing bias and privacy is not only a matter of compliance but also a reflection of an organisation’s values and commitment to social responsibility. By prioritising ethics, data scientists can help build a future where technology enhances human well-being and respects individuals’ rights.
BUSINESS DETAILS:
NAME: ExcelR- Data Science, Data Analyst, Business Analyst Course Training in Kolkata
ADDRESS: B, Ghosh Building, 19/1, Camac St, opposite Fort Knox, 2nd Floor, Elgin, Kolkata, West Bengal 700017
PHONE NO: 08591364838
EMAIL- enquiry@excelr.com
WORKING HOURS: MON-SAT [10AM-7PM]